SageMaker, by Amazon, is a managed service for machine learning that provides an array of tools to facilitate the building, training and deployment of machine learning models on a large scale.
Integrated development environment (IDE)
It encompasses an integrated development environment (IDE) equipped with features like notebooks, debuggers, profilers, pipelines and MLOps.
The key uses of SageMaker encompass constructing and training ML models, deploying models in a production environment, accessing and sharing code, accelerating AI modules, improving data training and inferences, optimizing data ingestion and output processes, handling datasets efficiently and supporting various industry specific use cases such as automotive applications, hospitality services, media and entertainment and pharmaceuticals among others].
Additionally, it offers advantages like enhancing productivity levels managing compute instances, automating the model creation deployment and training process with complete visibility.
From developing models to their deployment
In essence, Amazon’s SageMaker's primary purpose lies in streamlining the workflow of machine learning.
From model development up until its deployment.
Along with its extensive range of features that cater to different aspects of the machine learning lifecycle.
What are the advantages associated with employing Amazon Sagemaker for machine learning?
Amazon SageMaker brings forth benefits for machine learning projects, including:
Fully Managed Service; SageMaker efficiently handles the aspects of running a machine learning platform taking care of tasks like managing infrastructure.
This allows users to focus their attention on developing and deploying models.
One of its advantages is the range of algorithms and frameworks it provides access to.

It also offers support for custom algorithms giving developers the flexibility they need for model development.
SageMaker boosts productivity by accelerating machine learning development and streamlining the lifecycle with purpose-built tools.
Additionally, it excels at handling volumes of unstructured data making data preparation for machine learning easier and more efficient.
A notable benefit is that SageMaker is designed to be cost-effective with reduced cost of ownership (TCO) and lower data labeling costs.
Notably, it caters to diverse industry use cases such as automotive, hospitality, media and entertainment and pharmaceuticals among others, making it suitable for a range of domains.
In summary, Amazon SageMaker offers many benefits to building M/L. It manages major aspects effectively while supporting a variety of algorithms; it enhances productivity; it is cost-effective; and it can be applied across different industries.
What are some practical applications of Amazon Sagemaker in industries?
Amazon SageMaker is widely used across industries for a range of purposes.
Let's explore some of the use cases of Amazon SageMaker in sectors;
- Call Centers; Companies leverage SageMaker to develop machine learning models that help call center teams analyze customer interactions and enhance service efficiency.
- Manufacturing Sector; In this industry SageMaker plays a role in tasks like predictive maintenance, demand forecasting and ensuring quality control during the manufacturing processes.
- Hospitality; The hospitality industry benefits from SageMaker by utilizing it for enhancing customer experiences, demand forecasting and optimizing pricing strategies.
- Entertainment; In media and entertainment SageMaker proves valuable for content recommendation systems, video analysis, and creating personalized content.
- Pharmaceuticals; Within the pharmaceutical sector Amazon Sagemaker supports the drug development lifecycle.
- Data Analytics; Across industries, SageMaker is extensively employed for data analytics tasks such as data preparation, model training and inference.
- Earth Sciences; In the field of earth sciences researchers use SageMaker for climate modeling, predicting disasters and performing geospatial analysis.
These are a few examples highlighting how different industries make use of Amazon Sagemaker to address their specific needs.
How does Amazon Sagemaker contribute to cost reduction for businesses?
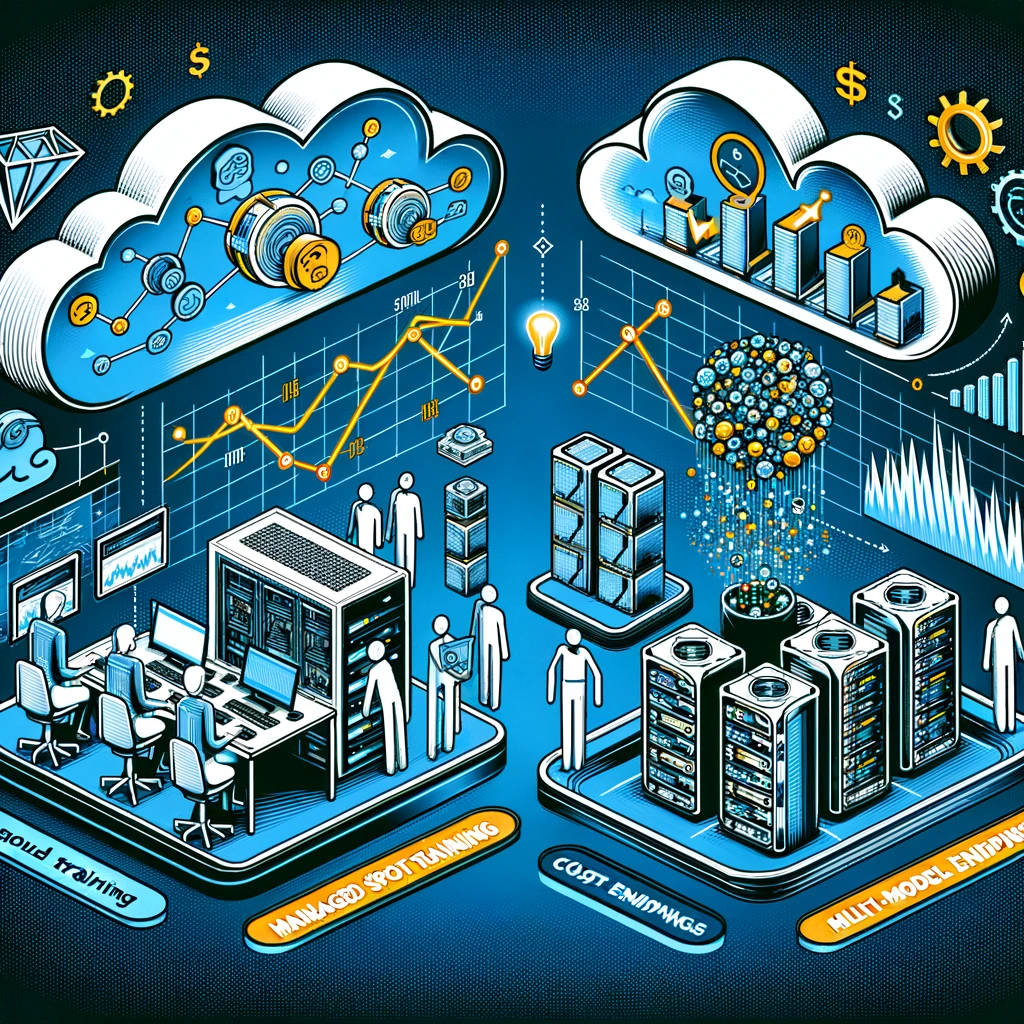
Amazon SageMaker provides options and capabilities to optimize costs, for companies using its robust features.
One of these cost optimization features is Managed Spot Training, which allows users to take advantage of EC2 capacity and effectively reduce the expenses associated with training machine learning models.
Another useful feature is the Multi-Model Endpoints, where you can host models on an endpoint. This helps optimize the utilization of deployed models and ultimately saves costs.
SageMaker also offers ML Savings Plans that contribute to reducing the cost of ownership (TCO) for ML workloads compared to self-managed alternatives like Amazon Elastic Compute Cloud (Amazon EC2).
With a 54% cost of ownership (TCO) and a 40% reduction in data labeling expenses compared to other options, Amazon SageMaker provides an affordable machine-learning solution.
Furthermore; it offers visibility and cost management tools that allow businesses to track, analyze, and optimize their SageMaker usage effectively for cost reduction.
Overall, Amazon SageMaker incorporates built-in options, for cost optimization, affordable solutions, and tools that improve visibility while enabling cost management.